AI in Environmental science marks a pivotal shift in how researchers and practitioners approach ecological challenges. AI, with its suite of computational and analytical tools, including machine learning (ML), natural language processing (NLP), and computer vision, has begun to alter the landscape of environmental research and conservation significantly. This section will explore the breadth and depth of AI’s role, showcasing its capacity to enhance data analysis, improve prediction accuracy, and foster innovative solutions to complex environmental issues.
1. AI in Environmental Science
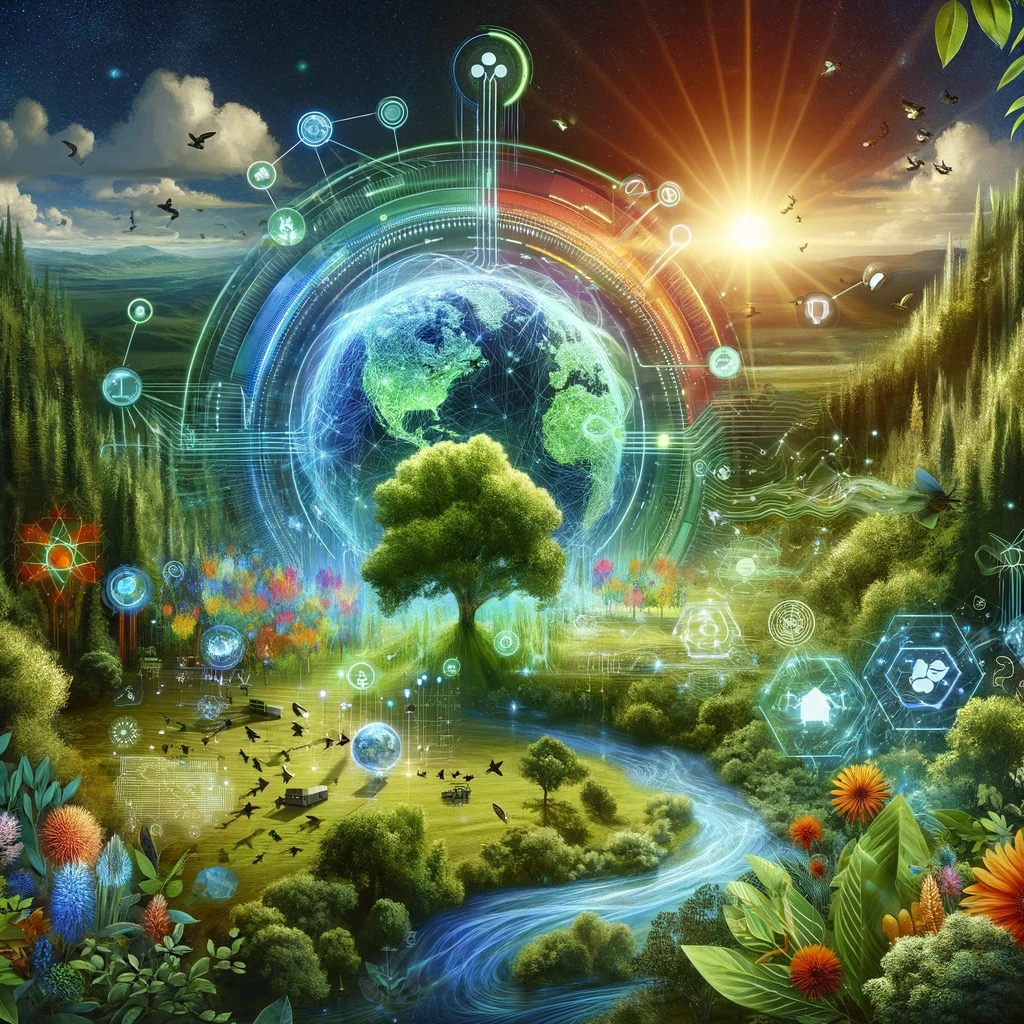
Enhancing Research and Conservation Efforts
AI technologies are revolutionizing environmental monitoring, data collection, and analysis processes.
By processing vast amounts of data from various sources—such as satellite images, sensor networks, and historical climate records—AI algorithms can identify patterns and anomalies that humans would be unable to discern unaided.
This capability accelerates research efforts and increases their accuracy, allowing for more precise forecasts and effective conservation strategies.
Machine learning models, a subset of AI, are particularly adept at predicting outcomes based on historical data.
In environmental science, this means improved forecasts of climate change impacts, more accurate tracking of wildlife populations, and better assessments of natural resource depletion.
These advancements are crucial for developing proactive measures to protect vulnerable ecosystems and species.
AI’s Role in Tackling Global Environmental Challenges
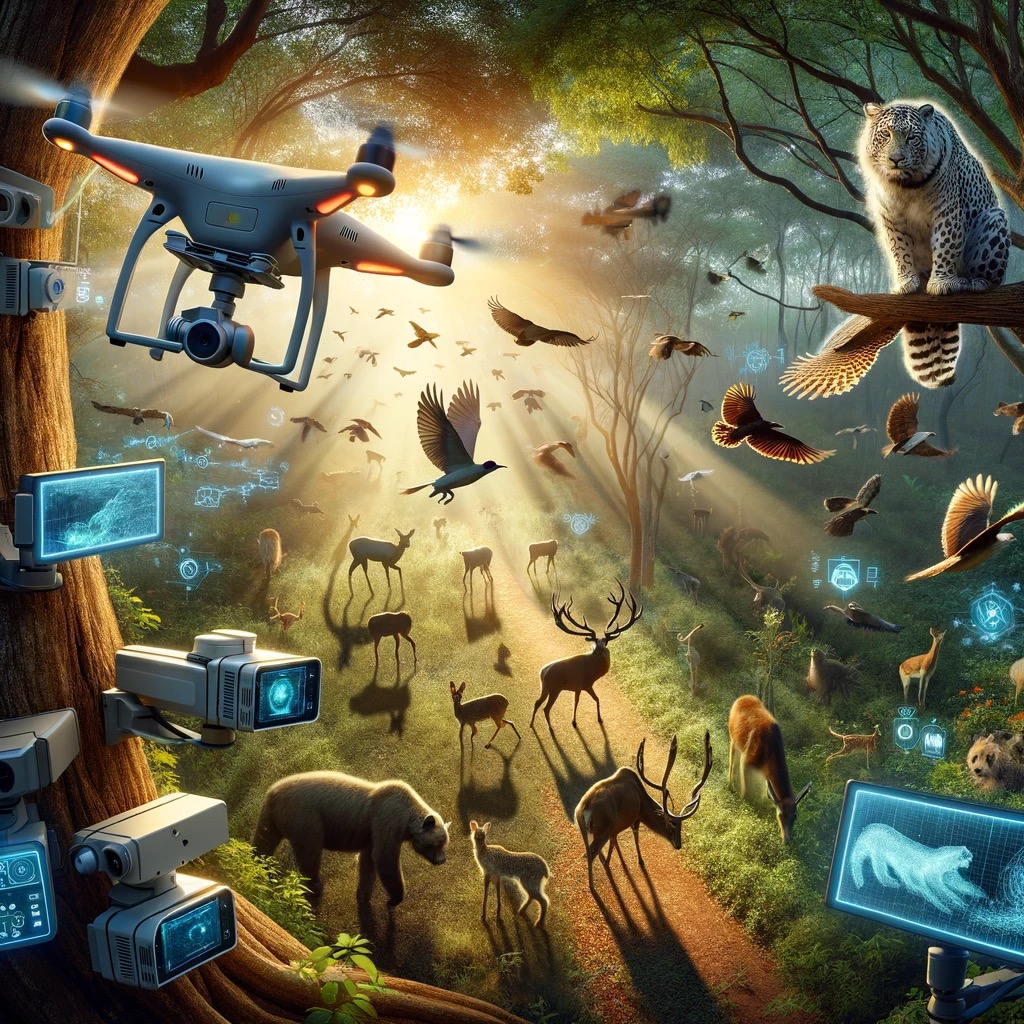
The application of AI extends across various domains within environmental science, each addressing critical global challenges:
- Climate Change: AI models enhance our understanding of climate dynamics and predict future climate patterns, contributing to more effective mitigation and adaptation strategies.
- Biodiversity Conservation: AI-driven tools facilitate monitoring species and habitats, helping protect biodiversity by identifying threats and informing conservation decisions.
- Pollution Control: AI algorithms are crucial in detecting pollution sources, monitoring air and water quality, and optimizing waste management practices.
- Sustainable Resource Management: AI supports the sustainable management of natural resources by optimizing usage, reducing waste, and predicting future demand.
Transformative Impact of AI
The transformative impact of AI on environmental science is profound. It offers a way to address the limitations of traditional research methods, such as the inability to process large datasets or the time required for manual data analysis.
AI’s predictive capabilities and real-time analysis enable more dynamic and responsive environmental management practices.
Furthermore, AI technologies facilitate interdisciplinary collaboration, bringing together experts from computer science, ecology, climatology, and other fields to tackle environmental issues innately.
This collaborative approach, enhanced by AI’s analytical power, leads to breakthroughs in understanding and preserving our natural world.
Future Prospects and Directions
As AI technologies continue to evolve, their role in environmental science is set to expand.
Future developments are likely to focus on improving the precision of AI models, enhancing the integration of AI with other technological advancements (such as IoT devices for real-time monitoring), and addressing the challenges of scalability and accessibility for global conservation efforts.
Moreover, as the ethical and practical implications of AI deployment become more apparent, there will be a greater emphasis on developing AI solutions that are not only effective but also sustainable and equitable.
This includes considerations around data privacy, algorithmic bias, and ensuring that AI technologies do not inadvertently harm the environments they protect.
AI’s integration into environmental science heralds a new era of research and conservation characterized by increased efficiency, accuracy, and innovation.
As we face global ecological challenges, AI is a beacon of hope, offering tools and technologies to help us better understand and protect our planet.
The ongoing collaboration between AI and environmental science promises to uncover new pathways to sustainability, making it an essential area of focus for researchers, policymakers, and conservationists.
2. AI in Climate Change Research
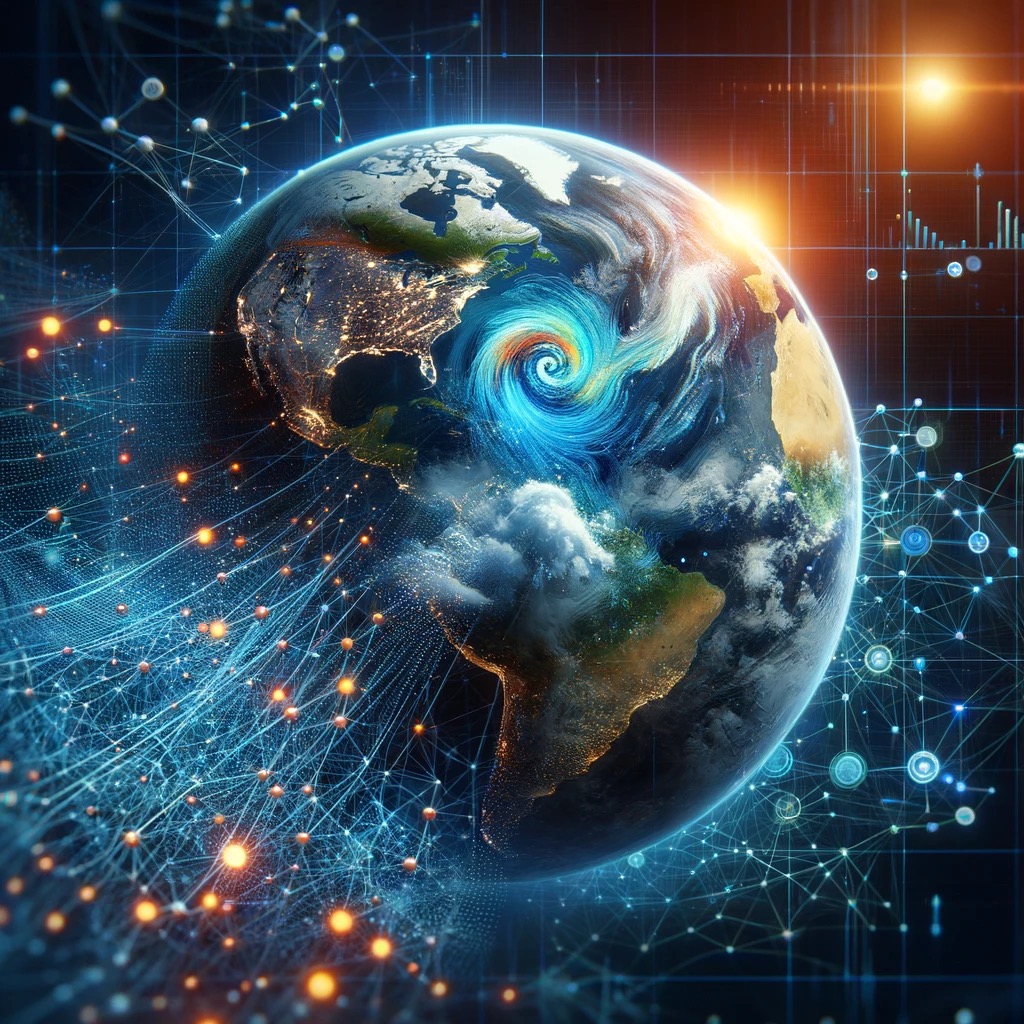
Climate change is a vast and complex challenge that requires innovative solutions to understand, mitigate, and adapt to its impacts.
Artificial Intelligence (AI) is at the forefront of this battle, offering new avenues for climate modeling, prediction, and action.
Utilizing AI for Climate Modeling and Prediction
AI, particularly machine learning (ML) and deep learning (DL) has significantly enhanced the accuracy and efficiency of climate models.
Traditional climate models, while informative, often need help with the sheer volume of variables and the complexity of interactions within the Earth’s climate system.
AI algorithms find patterns in large datasets, making them ideal for interpreting the data collected from satellite imagery, ocean buoys, weather stations, and other sources.
These AI-enhanced models can account for myriad factors influencing climate change, from greenhouse gas emissions to deforestation and urbanization, providing a more holistic and accurate picture of future scenarios.
One key advantage of AI in this context is its ability to process and analyze data in real-time.
This enables more dynamic and precise forecasting of weather patterns, temperature changes, and extreme climate events.
This capability is crucial for developing timely and effective disaster preparedness and risk management strategies.
Case Studies of AI in Understanding and Combating Climate Change
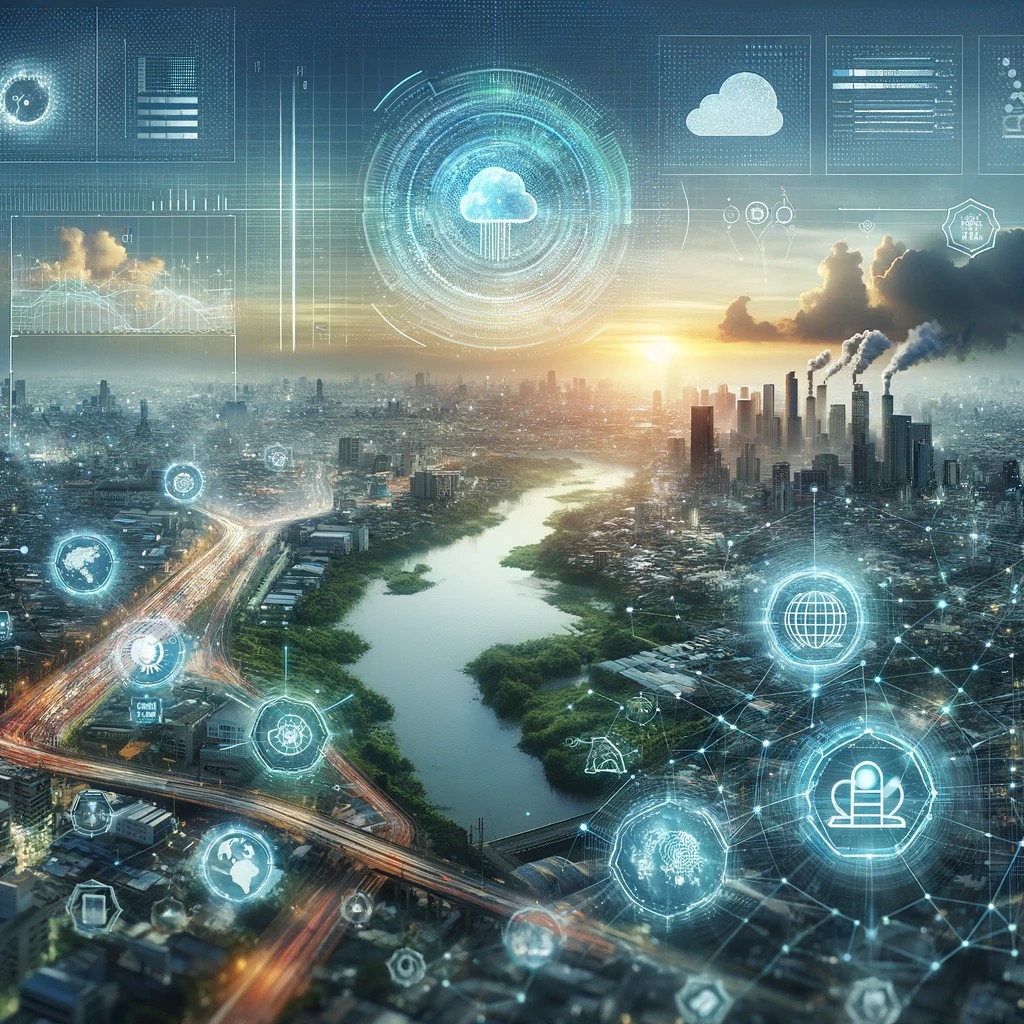
- Predicting Extreme Weather Events: Researchers are using AI to predict extreme weather events with greater accuracy and lead time than ever before. For example, AI models have been developed to forecast hurricanes, heat waves, and floods, allowing for earlier warnings and more effective emergency response strategies.
- Carbon Footprint Analysis: AI is instrumental in analyzing and reducing carbon footprints. Machine learning algorithms assess energy consumption patterns and optimize renewable energy use in real-time, significantly reducing greenhouse gas emissions. For instance, Google’s use of DeepMind AI to optimize data center cooling systems resulted in a 40% reduction in cooling energy consumption, demonstrating the potential of AI to enhance energy efficiency.
- Assessing Climate Change Impacts: AI models are being applied to assess climate change’s impacts on ecosystems and human societies. By analyzing satellite imagery and environmental data, AI helps identify areas at risk of climate-induced phenomena such as sea-level rise, desertification, and biodiversity loss. This information is vital for planning conservation efforts and adapting land use policies to mitigate adverse effects.
The Role of AI in Climate Action
Beyond research and modeling, AI is critical to climate action by informing policy decisions, enhancing public awareness, and driving innovations in sustainable technology.
AI-driven platforms analyze data from various sources to recommend the most effective interventions for reducing emissions, conserving natural resources, and promoting sustainable practices across industries.
AI also supports the development of smart grids and renewable energy systems, optimizing energy generation, distribution, and consumption to maximize efficiency and minimize waste.
Additionally, AI-powered solutions are emerging in areas like agriculture and transportation, where they help reduce the carbon footprint through precision farming and route optimization, respectively.
Challenges and Future Directions
While AI offers promising solutions for addressing climate change, challenges remain regarding data availability, model accuracy, and the ethical use of technology.
Ensuring the accessibility of high-quality, comprehensive datasets is crucial for developing effective AI models.
Moreover, ongoing research is needed to improve model interpretability and reliability, particularly in predicting long-term climate trends.
Integrating AI with other emerging technologies, such as blockchain for tracking carbon emissions or IoT devices for monitoring environmental conditions, presents exciting opportunities for advancing climate research and action.
As the field continues to evolve, the collaborative efforts of scientists, policymakers, and technologists will be vital to unlocking AI’s full potential in the fight against climate change.
AI’s contribution to climate change research is undeniable, offering new insights, enhancing predictive capabilities, and supporting actionable solutions to mitigate the effects of global warming.
As technology advances, the role of AI in understanding and combating climate change is set to grow, promising a future where data-driven decisions lead the way in safeguarding the planet for future generations.
3. AI in Wildlife and Biodiversity Conservation
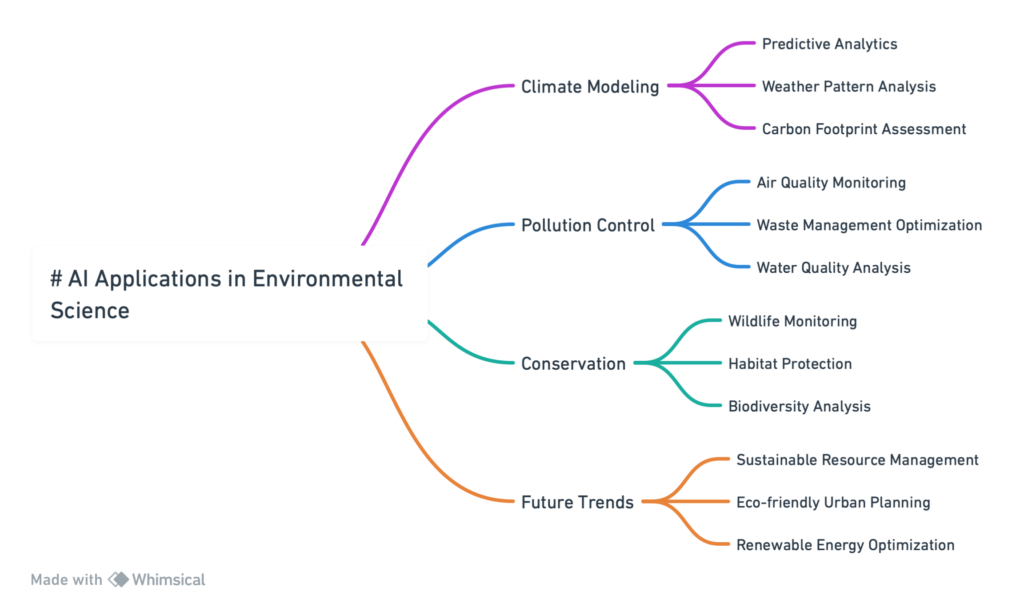
Preserving wildlife and biodiversity is crucial for maintaining ecological balance and ensuring the health of our planet.
Artificial Intelligence (AI) plays an increasingly significant role in conservation efforts, providing innovative tools for monitoring species, protecting habitats, and preventing biodiversity loss.
Role of AI in Wildlife Monitoring and Habitat Protection
AI technologies, particularly machine learning (ML) and computer vision have transformed how conservationists monitor wildlife populations and their habitats.
AI algorithms can identify, count, and track species across vast and often inaccessible areas by analyzing data from camera traps, drones, and satellite imagery.
This real-time monitoring capability is vital for understanding species behaviors, population dynamics, and threats they may face.
- Species Identification and Population Tracking: AI-powered camera traps and image recognition software enable the automatic identification and counting of individual animals within their natural habitats. This technology is beneficial for monitoring endangered species, allowing conservationists to gather accurate population estimates and observe trends over time without disturbing the animals.
- Habitat Protection: Satellite imagery analyzed with AI techniques can detect changes in land use, deforestation rates, and the encroachment of human activities into natural habitats. This information is crucial for implementing protective measures, restoring degraded ecosystems, and planning conservation strategies that ensure the sustainability of wildlife populations.
Examples of AI Applications in Conservation Efforts
- Predicting Poaching and Illegal Logging: AI models analyze human movement patterns and environmental conditions to predict poaching and illegal logging activities. These predictive insights enable law enforcement and conservation agencies to allocate resources more effectively and intervene before crimes against wildlife and nature occur.
- Acoustic Monitoring for Species and Ecosystem Health: Conservationists use AI to analyze audio recordings from natural habitats, identifying species-specific calls and sounds that indicate the presence and health of animal populations. This acoustic monitoring helps assess ecosystem health and detect disturbances that could signal environmental threats or illegal activities.
Innovations in Conservation Strategies
The integration of AI into conservation strategies has led to significant innovations:
- Drone Technology: Drones equipped with AI-powered cameras and sensors monitor large and difficult-to-access areas in real-time, providing valuable insights into wildlife movements, habitat conditions, and potential threats such as poachers or natural disasters.
- Genetic Analysis: AI aids in analyzing genetic data, helping researchers understand the genetic diversity within and across species. This information is crucial for breeding programs, species conservation efforts, and the restoration of gene health in at-risk populations.
Challenges and Opportunities
While AI offers transformative potential for wildlife and biodiversity conservation, there are challenges to its implementation, including the need for extensive data, the potential for bias in AI models, and the integration of AI insights into conservation policies and practices.
Addressing these challenges requires interdisciplinary collaboration, continued technological innovation, and the development of ethical guidelines for using AI in conservation.
The future of AI in this field is bright, with emerging technologies like deep learning and edge computing poised to enhance monitoring and protection efforts further.
By harnessing these advancements, conservationists can not only react to current threats but also anticipate and mitigate future challenges, ensuring the preservation of biodiversity for generations to come.
AI’s role in wildlife and biodiversity conservation is a testament to technology’s power to impact the natural world positively.
Through advanced monitoring, predictive analytics, and innovative conservation strategies, AI is helping to protect endangered species, preserve habitats, and maintain the rich diversity of life on Earth.
As we face environmental challenges, the responsible and innovative use of AI in conservation efforts represents a beacon of hope and a path toward sustainable coexistence with nature.
4. AI in Pollution Control and Management
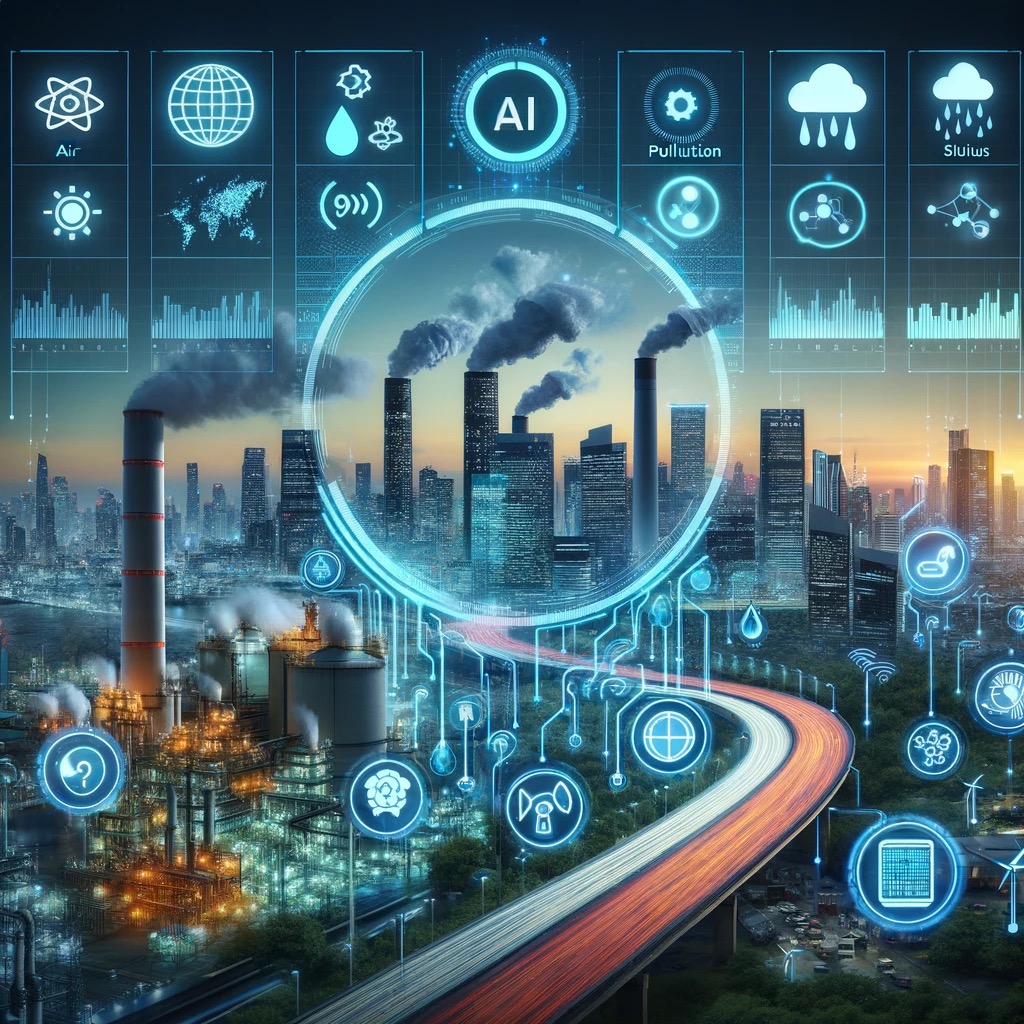
Pollution management and control are paramount in protecting environmental health and ensuring sustainable development.
Artificial Intelligence (AI) has emerged as a powerful tool for identifying, monitoring, and reducing pollution in air, water, and land.
AI-driven Solutions for Monitoring and Reducing Pollution
AI’s ability to process and analyze large datasets quickly and accurately makes it an invaluable asset in pollution control. Machine learning algorithms, combined with sensor data, satellite imagery, and other environmental data, provide a comprehensive view of pollution levels, sources, and trends.
- Air Quality Monitoring: AI systems analyze data from air quality monitoring stations to predict pollution levels, identify pollution sources, and assess the effectiveness of pollution control measures. These predictions are crucial for issuing warnings to the public, enforcing environmental regulations, and planning urban development to minimize air pollution.
- Water Quality Assessment: AI models process data from water monitoring sensors to detect contaminants, assess water quality in real time, and predict potential outbreaks of waterborne diseases. This information is vital for safeguarding public health, managing water resources, and protecting aquatic ecosystems.
Innovations in Waste Management and Air Quality Control
The application of AI in waste management and air quality control has led to several innovative solutions:
- Innovative Waste Management: AI-powered systems optimize waste collection routes, predict waste generation patterns, and automate the sorting of recyclables from waste streams. These innovations increase the efficiency of waste management processes and contribute to higher recycling rates and reduced landfill use.
- Predictive Air Quality Models: By combining AI with geographic information systems (GIS) and remote sensing data, researchers and policymakers can develop predictive models of air quality at both local and global scales. These models inform strategies for reducing emissions from key sources such as transportation, industry, and agriculture, improving air quality and public health.
- Pollution Source Identification: AI algorithms analyze patterns in environmental data to pinpoint pollution sources. Identifying these sources is critical for targeted interventions and for holding polluters accountable, thereby reducing pollution’s overall impact on the environment.
Case Studies Highlighting AI’s Impact
- Real-time Air Quality Monitoring in Urban Areas: In several cities worldwide, AI-driven air quality monitoring networks provide real-time data to residents and authorities, enabling timely actions to reduce exposure to harmful pollutants.
- AI for Reducing Plastic Pollution: Initiatives utilizing AI to monitor and manage plastic waste in oceans and rivers demonstrate how technology can be applied to tackle one of our most pressing environmental issues.
Challenges and Future Directions
While AI offers promising pollution control and management solutions, challenges such as data accuracy, privacy concerns, and the need for interdisciplinary collaboration must be addressed.
Ensuring the ethical use of AI and making technology accessible to communities worldwide are also crucial for maximizing its benefits.
The future of AI in pollution management holds the potential for even more sophisticated and integrated systems.
For instance, developing global pollution monitoring networks powered by AI could provide unprecedented insights into environmental health.
Additionally, integrating AI with other emerging technologies like blockchain for transparency in waste management or IoT devices for pervasive environmental sensing could further enhance pollution control efforts.
AI’s contribution to pollution control and management is a testament to its potential to address some of the most complex environmental challenges.
By harnessing the power of AI for monitoring, analysis, and intervention, we can make significant strides in reducing pollution and protecting the planet.
As we continue to innovate and integrate AI into environmental protection efforts, it is imperative to do so with a commitment to sustainability, equity, and the well-being of all living creatures.
5. AI in Sustainable Resource Management
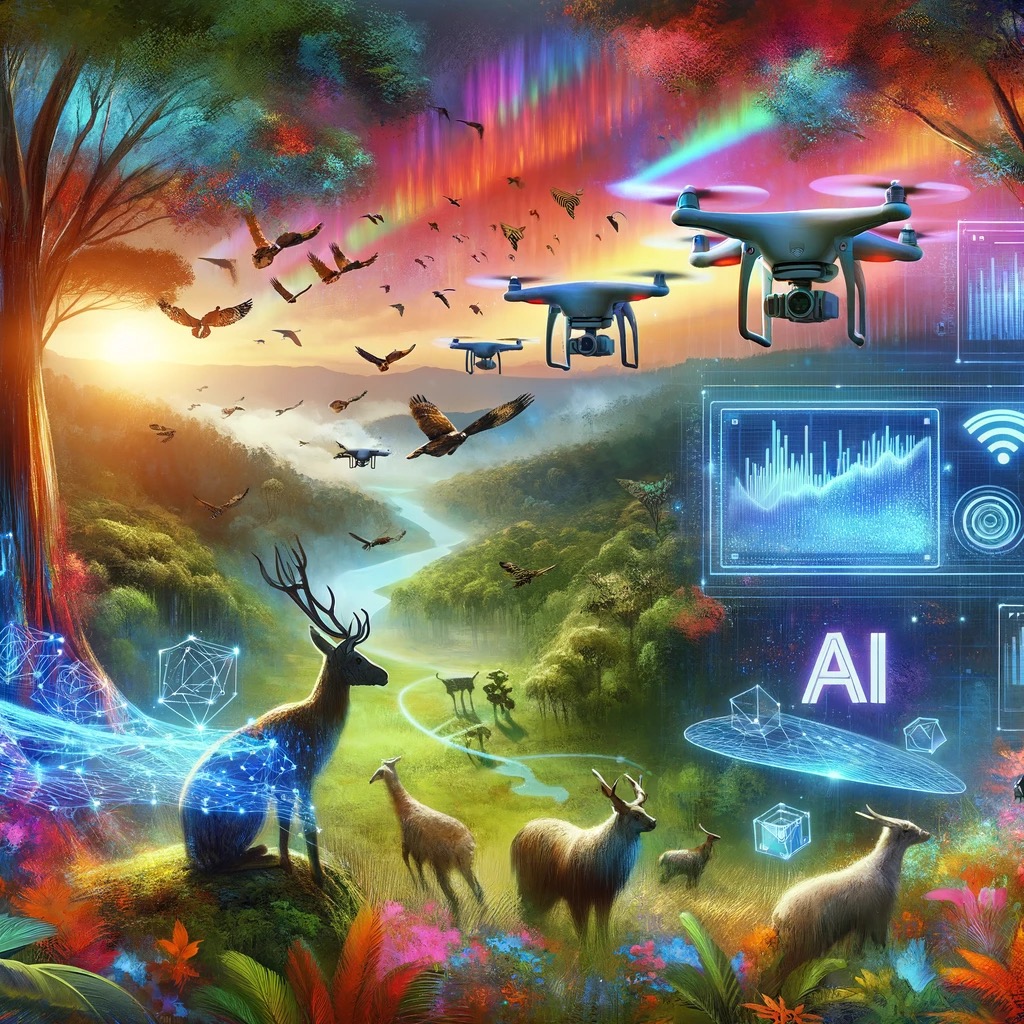
Sustainable management of natural resources is critical for preserving the environment, supporting economic growth, and maintaining quality of life.
Artificial Intelligence (AI) is increasingly recognized for its potential to enhance the sustainability of resource management practices across sectors such as agriculture, forestry, and water management.
Use of AI in Managing Natural Resources Sustainably
AI’s advanced data analysis and predictive capabilities enable more informed decision-making in natural resource management.
By leveraging machine learning algorithms, remote sensing data, and real-time monitoring systems, AI can optimize resource use, minimize waste, and predict future resource needs with unprecedented accuracy.
- Agriculture: AI technologies are revolutionizing agriculture through precision farming techniques. AI-driven systems analyze data from satellites, drones, and ground sensors to monitor soil health, crop growth, and water usage. This allows for precise application of water, fertilizers, and pesticides, reducing waste and environmental impact while maximizing crop yields.
- Forestry: In forestry, AI is used to monitor forest health, assess biomass, and predict fire risks. By analyzing satellite imagery and data from forest sensors, AI models can identify areas at risk of deforestation, pest infestations, or wildfires, facilitating timely interventions to sustainably protect and manage forest resources.
- Water Resource Management: AI aids in the sustainable management of water resources by predicting demand, optimizing distribution, and detecting leaks and inefficiencies in water supply systems. Machine learning models are also crucial in managing water quality, identifying potential contamination sources, and ensuring water supplies’ safety and sustainability.
AI in Agriculture, Forestry, and Water Resource Management
- Smart Irrigation Systems: AI-powered irrigation systems use data on soil moisture, weather forecasts, and crop needs to optimize watering schedules, significantly reducing water usage and improving water efficiency in agriculture.
- Forest Management and Restoration: AI applications in forestry include using drones and satellite imagery to monitor deforestation activities, assess tree species diversity, and plan reforestation projects, ensuring the ecological integrity and sustainability of forest ecosystems.
- Water Quality and Distribution: AI models that predict water quality and demand help plan and implement effective water distribution strategies, especially in regions facing water scarcity. These systems ensure that water resources are allocated efficiently, meeting the needs of populations while preserving natural water cycles.
Challenges and Opportunities
While AI offers significant benefits for sustainable resource management, challenges related to data accessibility, model accuracy, and the integration of AI into existing management frameworks must be addressed.
Additionally, resource managers and decision-makers must build capacity in AI technologies to fully leverage AI’s potential.
The future of AI in sustainable resource management is promising, with emerging technologies and approaches offering new opportunities for innovation.
For example, areas of growing interest are integrating AI with Internet of Things (IoT) devices for real-time resource monitoring and using blockchain for transparent and secure resource allocation.
AI’s role in sustainable resource management underscores its potential to contribute positively to environmental conservation and economic development.
By providing tools for precise resource monitoring, efficient usage, and predictive management, AI is helping to pave the way for a more sustainable and resource-efficient future.
As we continue to explore and expand the applications of AI in this field, it is crucial to do so with a focus on ethical considerations, equity, and the long-term well-being of both human and natural communities.
6. Challenges and Ethical Considerations
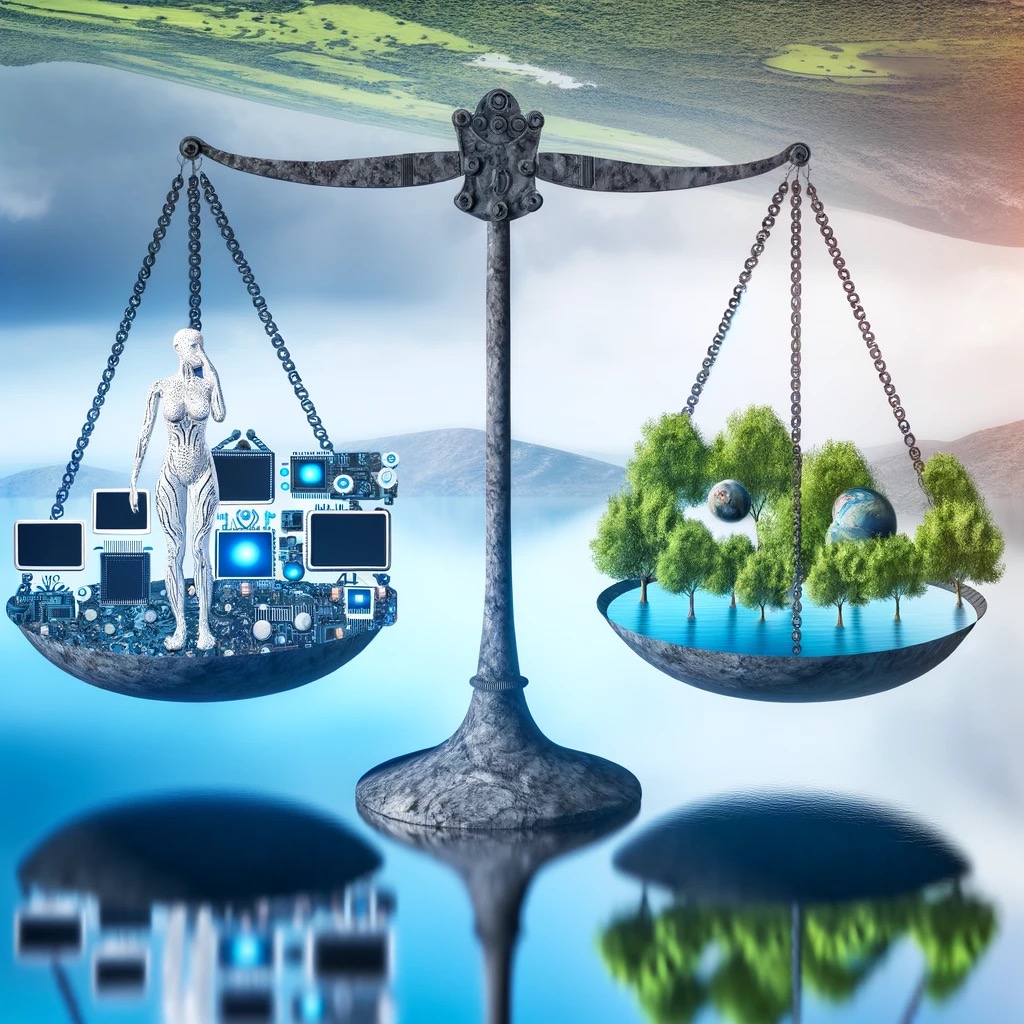
As Artificial Intelligence (AI) becomes increasingly integrated into environmental science and sustainability efforts, it brings transformative potential, challenges, and ethical considerations.
Responsible deployment of AI technologies is crucial to ensure they contribute positively to environmental goals without causing unintended harm.
Addressing the Ethical Implications of AI in Environmental Science
- Data Privacy and Security: The collection and analysis of environmental data through AI often involve sensitive information, raising concerns about data privacy and security. Ensuring the protection of this data against misuse or breaches is essential to maintain public trust and compliance with global data protection regulations.
- Bias and Fairness: AI systems are only as unbiased as the data on which they are trained. Historical biases in environmental data can lead to skewed AI models, potentially exacerbating existing inequalities in environmental protection and resource allocation. To ensure fair and equitable outcomes, efforts must be made to identify and correct these biases.
- Transparency and Accountability: AI models’ decision-making processes, especially those involving deep learning, can be opaque, making it challenging to understand how conclusions are reached. This lack of transparency complicates accountability, particularly in critical environmental decisions impacting communities and ecosystems.
Balancing Technological Intervention with Ecological Preservation
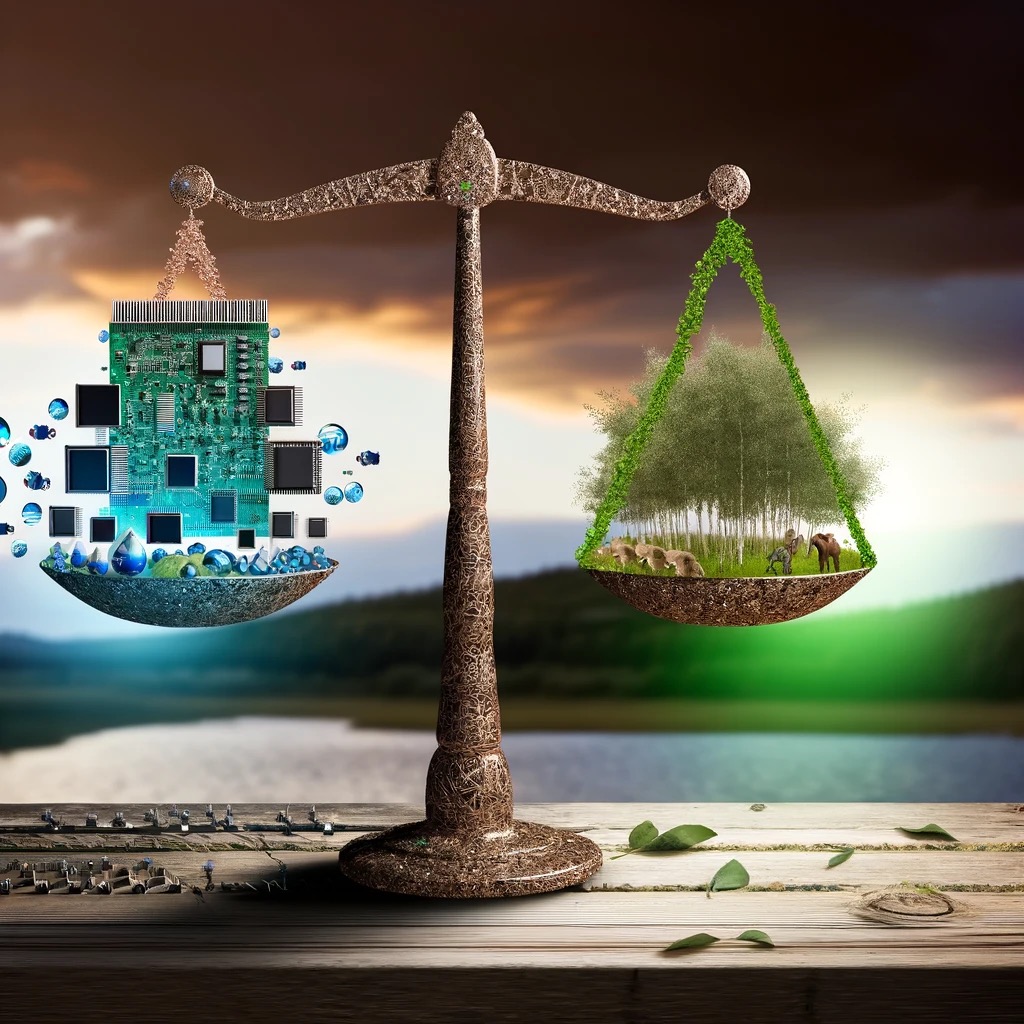
- Technological Overreliance: Heavy reliance on AI and technology could overshadow traditional conservation methods and indigenous knowledge that have been effective for centuries. Balancing modern technological interventions with conventional practices is crucial to ensuring comprehensive and culturally sensitive environmental solutions.
- Environmental Impact of AI Technologies: AI technologies have environmental footprints, primarily due to the energy consumption of data centers and the lifecycle impacts of hardware. Therefore, it is essential to consider and minimize the carbon footprint and resource use of AI systems deployed in environmental efforts.
Challenges in Implementation
- Scalability and Accessibility: While AI offers significant advantages, the scalability of AI solutions across different regions, especially in low-resource settings, remains challenging. Ensuring these technologies are accessible and adaptable to various environmental and socio-economic contexts is crucial for their global impact.
- Interdisciplinary Collaboration: Effective AI solutions for environmental challenges require collaboration across disciplines, including ecology, computer science, engineering, and social sciences. Developing holistic and impactful AI applications requires overcoming barriers to interdisciplinary cooperation.
Future Directions: Ethical AI for Environmental Science
- Developing Ethical Frameworks: Establishing ethical guidelines specific to using AI in environmental science is essential. These frameworks should address data governance, bias mitigation, transparency, and the ecological impact of AI technologies.
- Inclusive and Participatory Design: Involving communities, especially those most affected by environmental issues, in developing and implementing AI solutions can ensure that these technologies address real needs and do so ethically and fairly.
- Sustainability of AI Systems: Innovations in AI hardware and algorithms that reduce energy consumption and environmental impact are critical. Research into more efficient computing paradigms, such as neuromorphic and quantum computing, may offer paths forward.
Integrating AI into environmental science presents a complex landscape of opportunities and ethical challenges.
Navigating these challenges requires a careful and thoughtful approach, prioritizing sustainability, equity, and the well-being of both human and natural communities.
By addressing ethical considerations and focusing on responsible AI deployment, we can harness the power of AI to contribute significantly to environmental conservation and sustainability efforts, ensuring a balanced and respectful relationship with our planet.
7. The Future of AI in Environmental Science
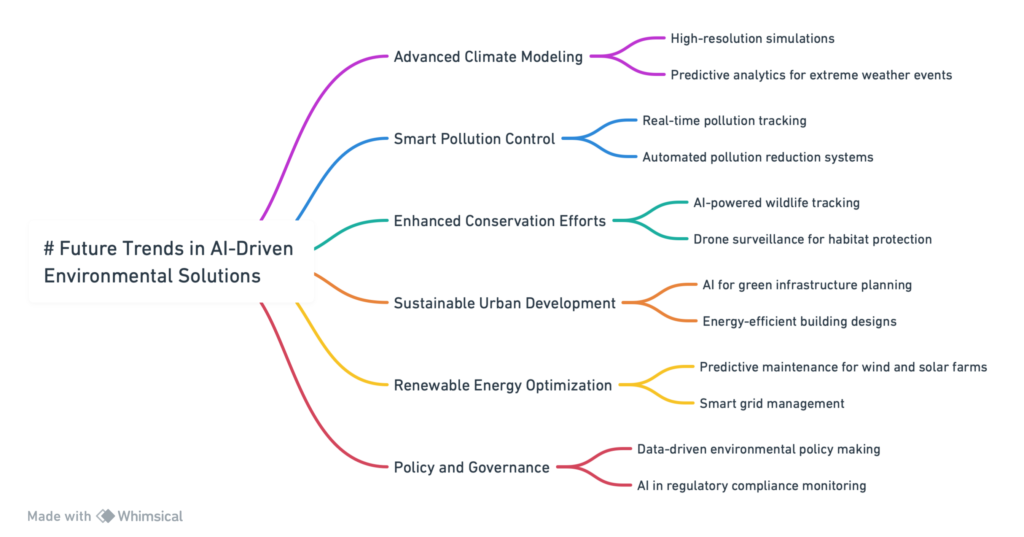
The role of Artificial Intelligence (AI) in environmental science is rapidly evolving.
It offers groundbreaking opportunities to enhance our understanding of the Earth’s systems and develop innovative solutions to pressing ecological challenges.
As we look to the future, AI has the potential to drive significant advancements in environmental protection and sustainability.
Predictions for AI’s Evolving Role
- Integrated Environmental Monitoring Systems: AI is expected to play a central role in developing integrated systems for real-time monitoring of environmental parameters worldwide. These systems will leverage satellite data, IoT devices, and ground-based sensors to provide unprecedented insights into climate change, pollution, biodiversity, and natural resource use.
- Advanced Climate Modeling: Future AI models will offer more accurate and granular predictions of climate patterns, extreme weather events, and their impacts on ecosystems and human societies. These advancements will be crucial for informing policy, planning, and adaptation strategies locally and globally.
- Precision Conservation: AI will enable more targeted and effective conservation efforts, identifying the most critical areas for intervention and predicting the outcomes of conservation actions. This precision approach will help optimize resource use and maximize the impact of conservation initiatives.
Emerging Trends and Future Directions
- AI for Sustainable Development: AI technologies align with the goals of sustainable development, focusing on creating solutions that promote environmental health, economic viability, and social equity. This includes AI applications in sustainable agriculture, clean energy, water conservation, and smart cities.
- Collaborative AI Platforms: The future will see the rise of collaborative AI platforms that bring together researchers, policymakers, and the public to share data, insights, and solutions. These platforms will foster a more inclusive and integrated approach to tackling environmental challenges.
- Ethical and Responsible AI Use: As AI becomes more embedded in environmental science, there will be an increasing focus on ensuring its ethical and responsible use. This includes addressing data privacy issues, algorithmic bias, and the ecological impact of AI technologies.
Challenges and Considerations for the Future
- Bridging the Digital Divide: Ensuring equitable access to AI technologies and their benefits is a critical challenge. Bridging the digital divide, particularly for communities in developing countries, will be essential to fully leveraging AI for global environmental sustainability.
- Interdisciplinary Research and Education: Advancing AI in environmental science will require continued investment in multidisciplinary research and education, which will train the next generation of scientists, engineers, and policymakers to work at the intersection of technology and environmental studies.
- Sustainability of AI Infrastructure: As AI systems become more sophisticated, their energy and resource demands will grow. Developing sustainable AI infrastructure, from energy-efficient data centers to eco-friendly computing technologies, will be paramount.
The future of AI in environmental science holds great promise, with the potential to revolutionize how we address and manage environmental challenges.
By harnessing AI’s power, we can unlock new insights into the Earth’s systems, develop more sustainable practices, and create innovative solutions to preserve the planet for future generations.
As we progress, navigating the ethical, technical, and social challenges accompanying these advancements will be crucial, ensuring that AI serves as a force for good in the quest for environmental sustainability.
8. Conclusion
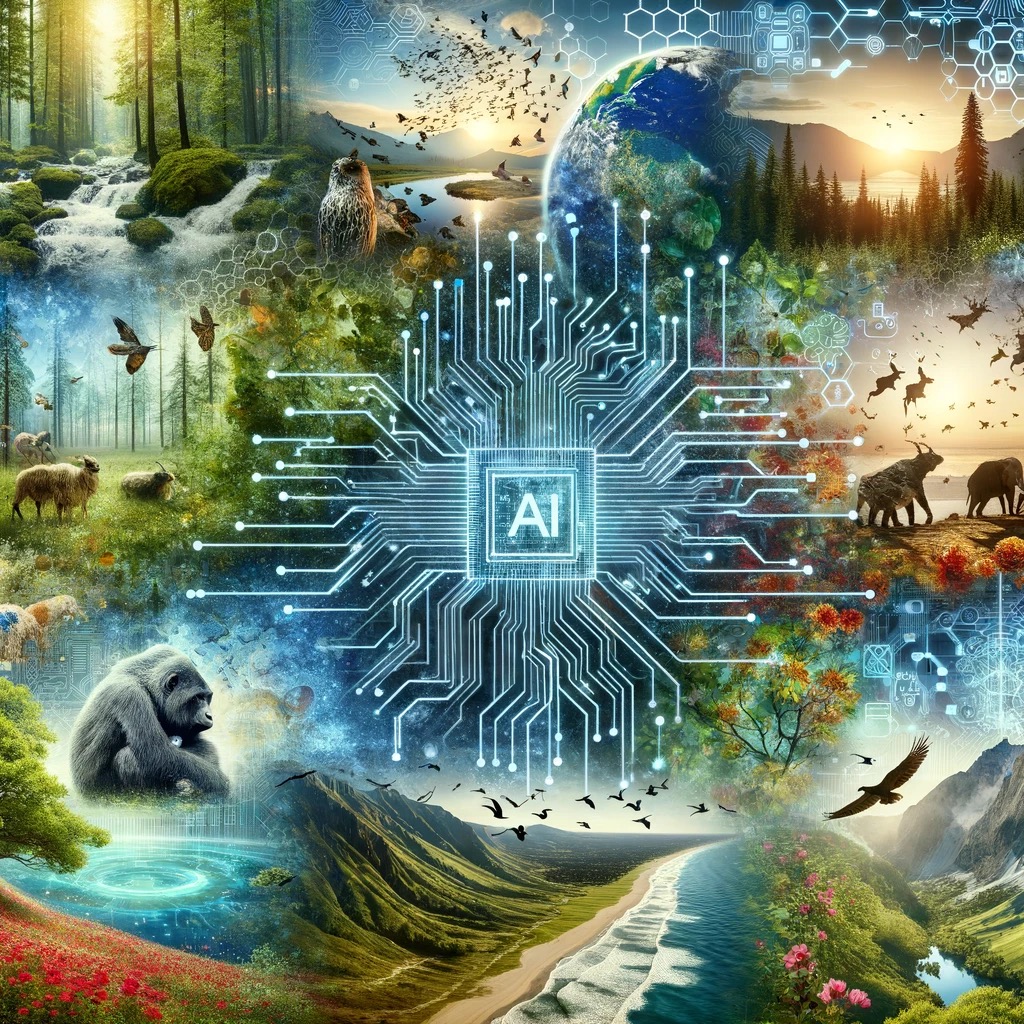
The exploration of Artificial Intelligence (AI) in environmental science has revealed its vast potential to revolutionize our approach to understanding, protecting, and managing the natural world.
From enhancing climate change research and wildlife conservation to innovating in pollution control and sustainable resource management, AI has demonstrated its capability to address some of our most pressing environmental challenges.
As we have navigated through the various facets of AI’s application in ecological science, several key points emerge that underscore the significance of this technological evolution.
AI offers transformative solutions that can accelerate the pace of research, increase the accuracy of environmental monitoring and predictions, and enable more efficient use of natural resources.
By leveraging machine learning, data analytics, and other AI technologies, scientists and conservationists can gain deeper insights into ecological processes, improve conservation strategies, and foster sustainable practices across industries.
Moreover, AI-driven pollution control and management innovations exemplify how technology can directly improve environmental health and human well-being.
However, integrating AI into environmental efforts is not without challenges and ethical considerations. Issues of data privacy, algorithmic bias, and the ecological impact of AI technologies themselves necessitate careful attention and responsible management.
The future of AI in environmental science hinges on our ability to navigate these challenges and ensure that AI is used ethically, sustainably, and equitably to benefit both the planet and its inhabitants.
AI’s role in environmental science is poised for further expansion and evolution. Emerging trends, such as integrating AI with other cutting-edge technologies and developing global collaborative platforms, promise to enhance our environmental protection and sustainability capabilities.
As we venture into this future, we must continue fostering interdisciplinary collaboration, investing in ethical AI research and development, and promoting inclusive access to AI technologies.
In conclusion, AI’s potential in advancing environmental science is both profound and indispensable.
By embracing responsible and innovative uses of AI, we can open new avenues for ecological research, conservation, and sustainability.
The journey ahead is filled with opportunities and challenges alike. Still, with a commitment to ethical principles and collaborative effort, AI can significantly contribute to the ecological well-being of our planet.
Encouraging the responsible use of AI in environmental science is not just an option—ensuring a sustainable and thriving future for all is imperative.
FAQ & Answers
1. How is AI used in environmental science?
AI is used for climate modeling, wildlife conservation, pollution control, and sustainable resource management, enhancing our understanding and preservation of the environment.
2. What are the challenges of implementing AI in environmental science?
Challenges include ethical considerations, ensuring accuracy in predictions, and balancing technological interventions with natural ecological processes.
Quizzes
Quiz 1: “AI in Environmental Science” – Identify AI applications in environmental scenarios.
Let’s explore how AI technologies are applied in various environmental science scenarios. Your task is to identify the specific AI application or technology being utilized in each situation:
- Wildlife Conservation: An organization uses drones equipped with cameras and AI to monitor wildlife in a protected area. The AI system analyzes video footage in real time to identify, count, and track different species, especially those that are endangered, helping in their conservation efforts.
- Climate Change Analysis: Researchers employ AI to process and analyze large datasets from satellite imagery, weather stations, and climate models. The AI system can detect patterns and predict future climate conditions, contributing to our understanding of climate change impacts and aiding in the development of mitigation strategies.
- Air Quality Monitoring: A city implements an AI-based system that uses data from sensors distributed across various locations to monitor air quality. The system analyzes pollutants in real-time and predicts air quality levels, providing valuable information to policymakers and the public for health and environmental planning.
- Water Quality Assessment: AI is used to analyze water samples from rivers and lakes, detecting contaminants such as heavy metals, bacteria, and chemicals. This system helps in identifying pollution sources and assessing water quality, ensuring safe drinking water and protecting aquatic ecosystems.
- Forest Fire Detection and Management: An AI-powered system analyzes satellite imagery and data from ground sensors to detect early signs of forest fires. The system can predict the spread of fires based on weather conditions and terrain, aiding firefighters and emergency responders in their efforts to control and extinguish fires more effectively.
- Sustainable Agriculture: Farmers use AI-driven tools to optimize crop yield and reduce environmental impact. The system analyzes data from soil sensors, weather forecasts, and satellite images to provide recommendations on irrigation, fertilization, and harvesting times, promoting more sustainable farming practices.
Identify the AI applications or technologies used in each of these environmental science scenarios.
Here are the AI applications or technologies being used in the environmental science scenarios provided:
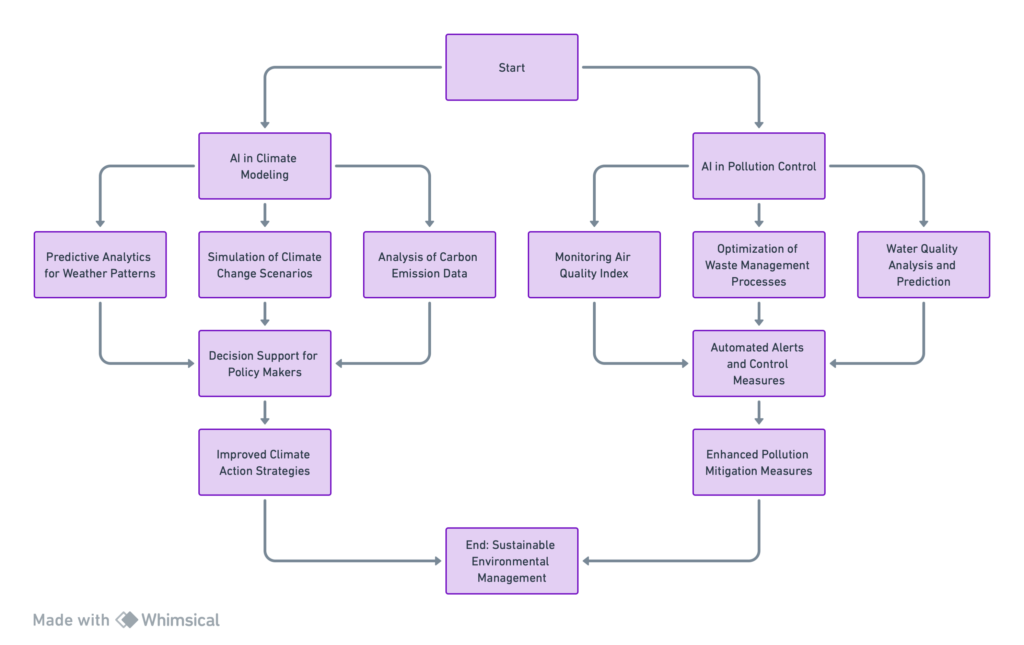
- Wildlife Conservation: The technology is AI-powered Image Recognition used in drones for wildlife monitoring. This application involves machine learning algorithms that analyze video footage to identify, count, and track various animal species, aiding in the conservation of endangered species and biodiversity.
- Climate Change Analysis: This scenario uses AI in Climate Modeling and Prediction. AI algorithms process vast amounts of data from satellites, weather stations, and climate models to identify patterns, predict future climate conditions, and help in understanding the impacts of climate change. This facilitates the development of effective mitigation and adaptation strategies.
- Air Quality Monitoring: The application is Real-time Air Quality Monitoring using AI. This system employs machine learning to analyze data from environmental sensors across a city to monitor pollutants and predict air quality levels, assisting in health and environmental policy making.
- Water Quality Assessment: Here, AI is applied in Water Quality Analysis and Pollution Detection. Machine learning algorithms analyze water samples for contaminants like heavy metals and bacteria. This technology helps in pinpointing sources of pollution and ensuring water safety for communities and ecosystems.
- Forest Fire Detection and Management: This involves AI for Early Fire Detection and Spread Prediction. Using data from satellites and ground sensors, AI systems detect early signs of forest fires and predict their spread, which is crucial for timely interventions by firefighters and emergency management teams.
- Sustainable Agriculture: The technology used is AI-driven Precision Agriculture. AI analyzes data from various sources, including soil sensors, weather forecasts, and satellite imagery, to optimize farming practices. Recommendations on irrigation, fertilization, and harvesting aim to maximize crop yield while minimizing environmental impacts, promoting sustainability in agriculture.
Each of these applications demonstrates the potential of AI to address critical environmental challenges, from conservation and climate change to pollution and sustainable agriculture, highlighting the role of technology in fostering a more sustainable and resilient future.
Quiz 2: “Eco-Innovations” – A quiz on recent AI innovations in environmental science.
For this quiz, I’ll describe some of the latest AI innovations in environmental science. Try to identify the specific innovation or the type of technology being discussed based on the descriptions:
- AI for Ocean Cleanup: This innovation involves deploying autonomous robots equipped with AI to identify and collect plastic waste from oceans and rivers. The robots are designed to navigate waters independently and distinguish between different types of marine debris, enabling more efficient cleanup efforts and reducing human labor.
- AI-enhanced Reforestation: Drones equipped with AI technology are used to map deforested areas and strategically drop seed pods in locations that maximize survival rates and biodiversity. The AI algorithms optimize reforestation efforts by analyzing terrain, soil conditions, and climate data to ensure the successful growth of new forests.
- Energy Consumption Optimization in Buildings: An AI system analyzes data from building sensors, weather forecasts, and energy prices to optimize heating, ventilation, and air conditioning (HVAC) systems in real-time. This reduces energy consumption and carbon emissions, contributing to more sustainable urban development.
- Predictive Maintenance for Renewable Energy Assets: AI algorithms predict when wind turbines and solar panels are likely to require maintenance or repair, based on data from sensors, historical performance, and weather conditions. This proactive approach minimizes downtime and maximizes the efficiency of renewable energy production.
- AI for Biodiversity Monitoring: This technology uses AI to analyze images and audio recordings from wilderness areas to monitor the presence and health of various species. It can identify changes in biodiversity and ecosystem health, alerting conservationists to potential environmental threats.
- Waste Sorting and Recycling Optimization: AI-powered systems in recycling facilities identify and sort waste materials more accurately and at a faster rate than human workers. This innovation improves recycling rates and the overall efficiency of waste management processes.
Identify the AI innovations or technologies used in these environmental science scenarios.
Here are the AI innovations or technologies being described in the environmental science scenarios:
- AI for Ocean Cleanup: The innovation is Autonomous AI-powered Robots for Marine Debris Collection. These robots use machine learning to identify, differentiate, and collect various types of plastics and other debris in water bodies, aiming to tackle the global issue of marine pollution efficiently.
- AI-enhanced Reforestation: This refers to AI-driven Drone Reforestation. Drones, guided by AI, map out deforested areas and strategically disperse seed pods to optimize reforestation efforts. AI algorithms consider various environmental factors to ensure high survival rates for new trees, contributing to ecosystem restoration and biodiversity conservation.
- Energy Consumption Optimization in Buildings: The technology is AI-based Smart Energy Management Systems for Buildings. These systems use AI to analyze multiple data streams and dynamically adjust HVAC operations, significantly reducing energy waste and carbon footprint while maintaining comfort levels within buildings.
- Predictive Maintenance for Renewable Energy Assets: This innovation involves AI-driven Predictive Maintenance for Wind Turbines and Solar Panels. By forecasting maintenance needs based on comprehensive data analysis, this technology ensures renewable energy assets operate at peak efficiency, reducing unexpected downtime and enhancing energy production.
- AI for Biodiversity Monitoring: The application is AI in Ecosystem Health and Biodiversity Monitoring. AI algorithms analyze environmental data, including images and sounds, to track the diversity and abundance of species. This technology provides insights into ecosystem changes, helping conservationists protect biodiversity.
- Waste Sorting and Recycling Optimization: This describes AI Systems for Automated Waste Sorting. In recycling facilities, AI-powered systems identify and sort recyclable materials with high accuracy and speed, improving the efficiency of recycling processes and supporting more sustainable waste management practices.
Each of these innovations showcases how AI can be leveraged to address critical environmental challenges, from protecting oceans and forests to optimizing energy use and enhancing biodiversity conservation, demonstrating the technology’s potential to support sustainable development and environmental protection.